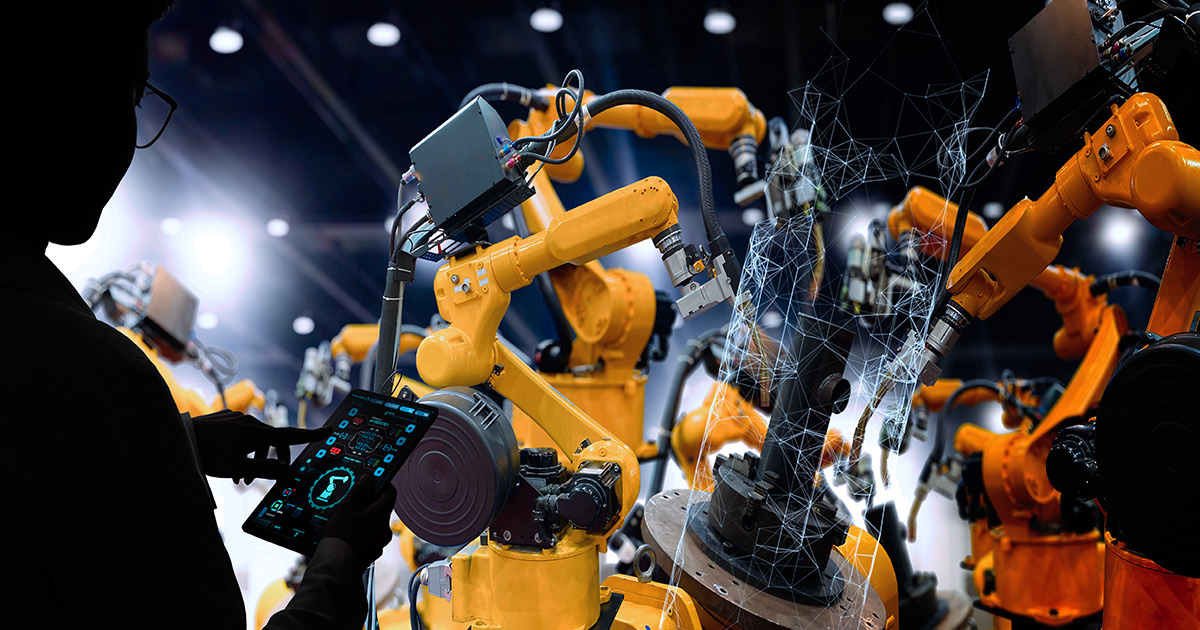
Let’s do an experiment: try writing “robots and jobs” in a search engine. In the summer of 2020, something like this comes out first. It mentions 51 million workers, in Europe alone, who risk their jobs within 10 years due to competition from robots. The source is a report by the consulting firm McKinsey published in mid-June, on the combined effects of automation and Covid-19 on the European labor market.
Just scroll through the search results, though, and you’ll find a different prediction just below. This time, it says that by 2037 automation and robotics will cancel 7 million jobs, but will also create 7.2 million new ones, for a total gain of 200,000 jobs. That sounds much better, you may say. But it depends on where your work is. If it's in manufacturing, and if you trust another Oxford Economics research from last year which you find a little further down in the search results, more jobs will disappear (20 million), and faster (by 2030).
We could go on, or do this experiment again in six months with different results. When it comes to predicting the future impact of robotics and artificial intelligence on the job market, choices abound: if this month's prediction seems too gloomy, all you have to do is wait for a better one next month.
“ It’s Difficult to Make Predictions, Especially About the Future Niels Bohr
A couple of years ago, the MIT Technology Review compiled a table with all the studies published on the subject, putting in a column the jobs to be destroyed and on another the jobs to be created according to each study (). The results range from the catastrophism of foreseeing 2 billion places destroyed worldwide by 2030, to the more optimistic but decidedly partisan vision of the International Federation of Robotics, that instead foresees 3.5 million places created thanks to robots by 2021. In between, all possible intermediate degrees of disruption.
Making predictions is hard, especially about the future, to quote a well-known aphorism often attributed to Niels Bohr (who in fact had at best borrowed it from someone else - in fact establishing true authorship of that phrase is even more difficult than predicting the future). If the future you are trying to predict concerns the economic and social impact of a new technology, it becomes even harder, and those who have tried in the past have mostly been proven wrong.
At the origin of the forecasts
The social relevance of this topic, however, is such that this field of study cannot be dismissed with a shrug. Artificial intelligence and robotics (especially the former) are progressing at a pace that seemed unthinkable a couple of decades ago, surpassing human performance in many areas. The possible consequences, should this progress result in mass unemployment, would threaten the very foundation of democracies. Studying future scenarios is necessary, in order to provide politicians with the elements to mitigate those consequences. But it's important to understand where data comes from, how scenarios are calculated, how credible they are.
In fact, the most-cited numbers come from three studies.
The study from Carl Frey and Michael Osborne
The first one is a 2013 study from the University of Oxford, by Carl Frey and Michael Osborne. It initially circulated as an internal document of the University and was published four years later, in a slightly revised form, in the journal Technological Forecasting and Change.
The most concise and most often cited result from this study is that "47 percent of jobs in the United States are at high risk of automation", and comes from the table below, which appeared in many news stories about the study:
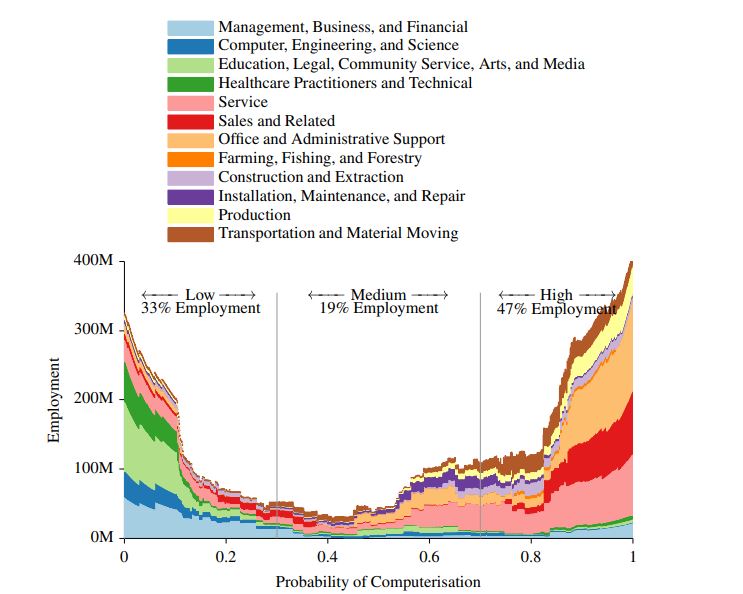
Each point of the table is a job (hairdresser, journalist, orchestra conductor, whatever), which can belong to one among 12 macro-sectors, identified by colors. Jobs on the left-hand side are less at risk, while those on the right hand side are more at risk. The height of the colored area tells you how many people are employed in that job according to the US statistical office. 33 percent of American workers therefore have jobs with a low risk of being replaced by a computer, 19 percent are at medium risk, while as many as 47 percent are at high risk of being replaced by an algorithm within a decade or two (and because the first study was from 2013, the first decade is already almost gone). The administrative, sales and services sectors are more at risk; health, finance and maintenance and installation of devices are safer.
Let's open the black box and see how the two researchers obtained their data. The first step was a workshop at the University of Oxford with the participation of "machine learning experts". We do not know how many or who they were, the paper does not say it and there is no trace of the workshop online (it must have taken place almost ten years ago, since the first study was in 2013). At any rate, Frey and Osborne gave to the workshop participants a list of 70 professions accompanied by an extended description. They took the information from O * net, an American database that encodes and standardizes information on occupations for statistical purposes.
For each of the 70 professions, Frey and Osborne asked the experts to answer the question: "Can the tasks required by this job be specified clearly enough to be performed by a computer?" In short, can you transform that job into an algorithm? The experts had to answer 0 if they considered that job not automatable, 1 if definitely automatable. The 70 jobs had been chosen in such a way that the answer was easy: surgeon, event organizer, stylist (all 0); dishwasher, telemarketing operator, court clerk (all 1). The two authors had also asked the experts about the main bottlenecks that artificial intelligence and robotics have yet to overcome, concluding that there are three of them: perception and manipulation, creative intelligence, social intelligence.
“ If a job requires these 9 high-grade skills, it's harder to automate Carl Frey and Michael Osborne
Second step: the two authors took the complete description of those 70 jobs in the O * net database, which for each job also includes a long list of cognitive and manual skills and knowledge needed to do it, and an index that says how much you have to be good at that thing to do that job. Then, among the dozens and dozens of variables that the database lists, they chose 9 that in their opinion best describe those three bottlenecks seen above: persuasion, negotiation, social sensitivity, originality, ability to deal with others, knowledge of fine arts, manual dexterity, ability to work in a cramped environment. The idea of the two authors is: if a job requires these 9 skills to a high degree, it is more difficult to automate.
Finally, Frey and Osborne used... an algorithm, of all things, to do a piece of work for them, and learn to estimate the probability of automation of a job starting from the importance that the O* net database assigns to those nine skills. They used the 70 jobs analysed in the workshop as a training set, to extract correlations between the probability of automation of a job and the scores it has on those 9 parameters. Then they extended the calculation to all 702 jobs of the complete list, obtaining for each a value between 0 and 1. In practice, they used the 70 "easy" jobs to teach the algorithm to predict how much a job can be automated based on how much requires those 9 skills, and then they made it calculate the risk for the other 630 jobs, the less easy ones. The complete data consists of a ranking of all occupations according to their automation risk, on a scale between 0 and 1. At the bottom, with the highest risk, you find jobs as a watch repairer, insurance agent, sewer, telemarketing operator, all with a score of 0.99. At the top, recreational therapist, mechanical machinery installers and maintainers, director of emergency procedures, all below 0.003.
From the "occupation-based" to the "task-based" approach: the OECD study
Frey and Osborne's process is ingenious, but also subjective and arbitrary. The choice of experts to be invited to the workshop is arbitrary; the choice of the 70 works to be submitted for their evaluation is arbitrary; the evaluation by those experts of which jobs are more or less automatable is subjective; the choice of those 9 skills, among the dozens required for a job, as those that best represent technological bottlenecks is extremely arbitrary.
The method is also very hard to replicate: anyone who, a few years after the original study, wished to update it should convene another workshop. And because it is not known who participated in the first one, the results would probably be different.
Another well-known study by the Organization for Economic Cooperation and Development (OECD) from 2016, as an explicit response Frey and Osborne’s catastrophism. And indeed, the conclusion here is less alarming: just 9 per cent of the jobs in the organisation's member countries are described as automatable, although with important regional differences: for example 6 percent of jobs in South Korea are automatable, contrasted with 12 percent in Austria.
In contrast with Frey’s and Osborne’ "occupation-based" approach, the OECD uses a "task -based ” one, where the tasks actually performed by individual workers are at the center of the analysis, rather than the whole occupation. Jobs are bundle of tasks, and if some of the crucial tasks in a job are purely "human", and if it is difficult to separate them from the others, in fact the employer will not be able to replace the worker with an algorithm, even though the rest of the tasks could be done by a robot. It is this subtlety that Frey and Osborne ignore and that the authors of the OECD report want to describe. To do this, they start from another statistical resource, the PIAAC database, a survey conducted in all OECD countries to measure the basic skills of the adult population between 16 and 65 years. The PIAAC survey uses cognitive tests and a questionnaire that documents education and training paths, professional history, employment status, skills used for one's job.
The OECD study uses a rather complex statistical method. Briefly, let's just say that the authors start from the automation risk assigned by Frey and Osborne to each occupation. they proceed to associate that number to each individual present in the PIAAC census, based on his declared occupation. They then use a probabilistic method to determine how much each of the actual tasks and skills described by the workers contribute to that risk. Assigning a weight to every single task, they recalculate everything, worker by worker, arriving at a very different result. In practice, the OECD study replaces those 9 variables used by Frey and Osborne to calculate the automation risk with a much longer and more complete list, and develops an algorithm that calculates the risk for each individual worker, and not for an abstract list of occupations. Unfortunately, this study, like the previous one, does not make all the data available to try to replicate the calculations.
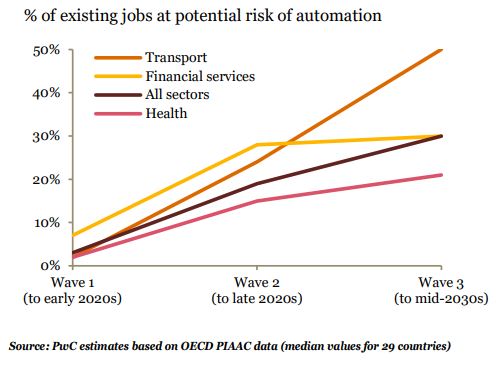
Half of the tasks are automatable: the McKinsey study
A third highly cited study is from 2017, and comes from McKinsey. This firm has produced several reports on automation and the future of work over the years, but the methodology is more or less always the same, inaugurated in "A future that works" (https://www.mckinsey.it/idee/harnessing-automation-for-a-future-that- works). McKinsey too, like the OECD study, chooses not to look at the jobs, but rather at the different activities that make them up. “For example,” the report writes “a salesperson will spend a certain amount of time interacting with customers, filling the shelves, recording sales. Each activity is different and requires different skills to be completed”.
McKinsey analysts also rely on the O *net database to break down existing professions into a list of 2,000 businesses. Then, always adapting the categories used by O * net, they draw up a list of 18 “requirements” (divided between sensory, cognitive, linguistic, social, physical capacities), and define each activity as a combination in different degrees of those 18 requirements. The diagram below may help:
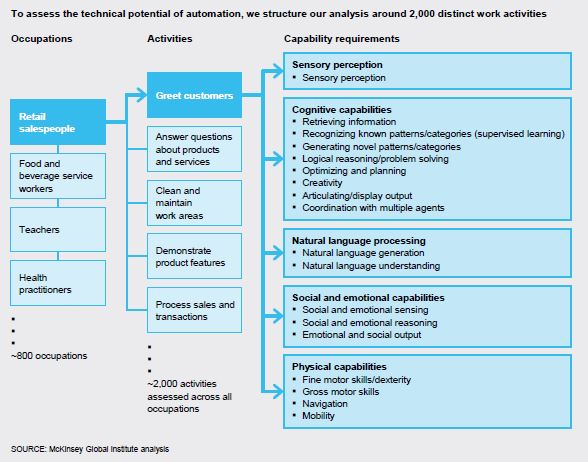
For each activity in the central column, the authors assign a score from 0 to 3 to each of the 18 requirements in the right-hand column, which expresses how important that capability is in order to carry out that activity. Finally, they estimate the technological feasibility of those 18 capabilities (that is, whether machines today possess them at levels comparable to humans, or if they will be able to have them in the near future) and go backward with the calculations. If an activity mostly requires capabilities that are still unattainable for machines, it is less automatable, otherwise it is more. This is more or less the Frey and Osborne method, but with more variables (18 instead of 9) and focusing not on the professions as a whole but on the activities of which they are composed (as recommended by the OECD). The results are more complex and nuanced than those of the other two studies.
According to McKinsey, almost half of the activities that make up today's jobs, worldwide, are potentially automatable. Less than 5 percent of professions are fully automatable, about 60 percent are composed of at least 30 percent of automatable activities. The automatable activities concern 1.2 billion workers worldwide and 14.6 million billion dollars in wages.
Poor clarity
The problem is that we know very little about how McKinsey's analysts went through each step. In the study there is not even a complete list of the 2000 "activities" used in the analysis, and we do not know who and how determined whether, for example, the activity "demonstrating product characteristics" requires creativity or not, and whether it requires it in grade 1, 2 or 3. As for the core of the analysis, ie the estimation of how much machines can compete with humans on those 18 abilities, the authors just tell us that:
“We used survey findings, extrapolation of metrics, and other predictors of technical advances. We conducted interviews with industry leaders and academic experts. We also looked at some recent commercial successes showcasing capabilities, as well as historic trajectory of capabilities. We then adjusted the result using some identified constraints that could limit the progression of certain capabilities.
To improve accuracy, we looked at other predictors, including from the press, companies, research groups, and relevant industries. These predictors include research and technological breakthroughs, trends in publications, and patents as a measure of research potential.
Based on the assessment, we projected the reasonable expected time frames to reach the next level of performance for each capability”.
All rather ... opaque, and hardly anyone could try to replicate the data. When we read the very interesting scenarios on the future of work designed by McKinsey in this and other subsequent reports, we must basically trust them. All of this is perfectly understandable for a private company that is not required to publish all the details of its work and indeed maintains a degree of industrial secrecy. The McKinsey report is not an academic paper, it must not provide the means to replicate the experiment. The problem is that academic or institutional studies are only slightly more transparent, do not make all the data available and are based on arbitrary and subjective evaluations.
In short, to date it is very difficult to indicate an authentically scientific study, which links current employment data with the state of the art of robotics and artificial intelligence and which allows anyone to verify and replicate the data.